Diurnal Change of NDVI from UAV in Trees of a Temperate Unavenged Forest Stand
DOI:
https://doi.org/10.29298/rmcf.v15i82.1431Palabras clave:
Spectral Indices, Individual Trees, Drones, Vigor, Uneven AgedResumen
NDVI elucidates the ecophysiological mechanisms faced by vegetation. With the flexibility and versatility of unmanned aerial vehicles (UAVs), temporal, spatial and spectral resolutions have been useful in supporting decision-making. Here, we modeled diurnal change in the NDVI derived from UAV imagery for individual trees in a natural forest stand. Eight flights were conducted during daylight hours over one day to assess the dynamics of the NDVI of the genera Pinus, Juniperus, and Quercus. The results showed unstable NDVI values over time, with a parabolic quadratic trend in the model. The NDVI reached its maximum around 13:00 h and the values differed significantly according to genus: the highest value was found in Pinus with significant differences presented with Juniperus and Quercus, which showed similar values between them (p=0.533). As a validation strategy, we test the model generated using 124 trees independent of those that were sampled, which strengthened our results in terms of reliability. The similarities of statistical parameters confer a high variability of application of the results in models of simulation of similar forests ecosystems. We recommend to study more spectral indices of vegetation, including calibration of the sensor, particularly in longer-term seasonal studies. We conclude that the NDVI measured using UAV should consider image acquisition time to calibrate the records and thus improve the interpretation of results.
Descargas
Citas
Beneduzzi, H. M., E. G. Souza, C. L. Bazzi and K. Schenatto. 2017. Temporal variability in active reflectance sensor-measured NDVI in soybean and wheat crops. Engenharia Agrícola 37(4):771-781. Doi: 10.1590/1809-4430-Eng.Agric.v37n4p771-781/2017. DOI: https://doi.org/10.1590/1809-4430-eng.agric.v37n4p771-781/2017
Buchhart, C. and U. Schmidhalter. 2022. Daytime and seasonal reflectance of maize grown in varying compass directions. Frontiers in Plant Science 13:1029612. Doi: 10.3389/fpls.2022.1029612. DOI: https://doi.org/10.3389/fpls.2022.1029612
Dronova, I. and S. Taddeo. 2022. Remote sensing of phenology: Towards the comprehensive indicators of plant community dynamics from species to regional scales. Journal of Ecology 110(7):1460-1484. Doi: 10.1111/1365-2745.13897. DOI: https://doi.org/10.1111/1365-2745.13897
Fawcett, D., J. Bennie and K. Anderson. 2021. Monitoring spring phenology of individual tree crowns using drone-acquired NDVI data. Remote Sensing in Ecology and Conservation 7(2):227-244. Doi: 10.1002/rse2.184. DOI: https://doi.org/10.1002/rse2.184
Gallardo-Salazar, J. L. and M. Pompa-García. 2020. Detecting individual tree attributes and multispectral indices using Unmanned Aerial Vehicles: Applications in a Pine clonal orchard. Remote Sensing 12(24):4144. Doi: 10.3390/rs12244144. DOI: https://doi.org/10.3390/rs12244144
Ghebrezgabher, M. G., T. Yang, X. Yang and T. E. Sereke. 2020. Assessment of NDVI variations in responses to climate change in the Horn of Africa. The Egyptian Journal of Remote Sensing and Space Science 23(3):249-261. Doi: 10.1016/j.ejrs.2020.08.003. DOI: https://doi.org/10.1016/j.ejrs.2020.08.003
González-Elizondo, M. S., M. González-Elizondo, J. A. Tena-Flores, L. Ruacho-González e I. L. López-Enríquez. 2012. Vegetación de la Sierra Madre Occidental, México: una síntesis. Acta Botánica Mexicana 100:351-403. Doi: 10.21829/abm100.2012.40. DOI: https://doi.org/10.21829/abm100.2012.40
Gujarati, D. N. and D. C. Porter. 2009. Basic Econometrics. McGraw-Hill. New York, NY, United States of America. 922 p.
Hama, A., K. Tanaka, B. Chen and A. Kondoh. 2021. Examination of appropriate observation time and correction of vegetation index for drone-based crop monitoring. Journal of Agricultural Meteorology 77(3):200-209. Doi: 10.2480/agrmet.D-20-00047. DOI: https://doi.org/10.2480/agrmet.D-20-00047
Heinemann, P., S. Haug and U. Schmidhalter. 2022. Evaluating and defining agronomically relevant detection limits for spectral reflectance-based assessment of N uptake in wheat. European Journal of Agronomy 140:126609. Doi: 10.1016/j.eja.2022.126609. DOI: https://doi.org/10.1016/j.eja.2022.126609
Huang, S., L. Tang, J. P. Hupy, Y. Wang and G. Shao. 2021. A commentary review on the use of normalized difference vegetation index (NDVI) in the era of popular remote sensing. Journal of Forestry Research 32(1):1-6. Doi: 10.1007/s11676-020-01155-1. DOI: https://doi.org/10.1007/s11676-020-01155-1
Jacquemoud, S., W. Verhoef, F. Baret, C. Bacour, … and S. L. Ustin. 2009. PROSPECT+SAIL models: A review of use for vegetation characterization. Remote Sensing of Environment 113(S1):S56-S66. Doi: 10.1016/j.rse.2008.01.026. DOI: https://doi.org/10.1016/j.rse.2008.01.026
Khaliq, A., L. Comba, A. Biglia, D. R. Aimonino, M. Chiaberge and P. Gay. 2019. Comparison of satellite and UAV-based multispectral imagery for vineyard variability assessment. Remote Sensing 11(4):436. Doi: 10.3390/rs11040436. DOI: https://doi.org/10.3390/rs11040436
Li, D., J. M. Chen, X. Zhang, Y. Yan, … and W. Cao. 2020. Improved estimation of leaf chlorophyll content of row crops from canopy reflectance spectra through minimizing canopy structural effects and optimizing off-noon observation time. Remote Sensing of Environment 248:111985. Doi: 10.1016/j.rse.2020.111985. DOI: https://doi.org/10.1016/j.rse.2020.111985
Li, W., J. Jiang, M. Weiss, S. Madec, … and F. Baret. 2021. Impact of the reproductive organs on crop BRDF as observed from a UAV. Remote Sensing of Environment 259:112433. Doi: 10.1016/j.rse.2021.112433. DOI: https://doi.org/10.1016/j.rse.2021.112433
Mamaghani, B. and C. Salvaggio. 2019. Multispectral sensor calibration and characterization for sUAS Remote Sensing. Sensors 19(20):4453. Doi: 10.3390/s19204453. DOI: https://doi.org/10.3390/s19204453
Maresma, A., L. Chamberlain, A. Tagarakis, T. Kharel, … and Q. M. Ketterings. 2020. Accuracy of NDVI-derived corn yield predictions is impacted by time of sensing. Computers and Electronics in Agriculture 169:105236. Doi: 10.1016/j.compag.2020.105236. DOI: https://doi.org/10.1016/j.compag.2020.105236
Pompa-García, M., J. A. Martínez-Rivas, R. D. Valdez-Cepeda, C. A. Aguirre-Salado, … and D. J. Vega-Nieva. 2022. NDVI values suggest immediate responses to fire in an uneven-aged mixed forest stand. Forests 13(11):1901. Doi: 10.3390/f13111901. DOI: https://doi.org/10.3390/f13111901
Prăvălie, R., I. Sîrodoev, I.-A. Nita, C. Patriche, … and M.-V. Birsan. 2022. NDVI-based ecological dynamics of forest vegetation and its relationship to climate change in Romania during 1987-2018. Ecological Indicators 136:108629. Doi: 10.1016/j.ecolind.2022.108629. DOI: https://doi.org/10.1016/j.ecolind.2022.108629
R Core Team. 2021. R: A Language and Environment for Statistical Computing (version 4.1.1.). R Foundation for Statistical Computing. Vienna, W, Austria. https://www.R-project.org. (November 6th, 2023).
Rischbeck, P., P. Baresel, S. Elsayed, B. Mistele and U. Schmidhalter. 2014. Development of a diurnal dehydration index for spring barley phenotyping. Functional Plant Biology 41(12):1249-1260. Doi: 10.1071/FP14069. DOI: https://doi.org/10.1071/FP14069
Vivar-Vivar, E. D., M. Pompa-García, J. A. Martínez-Rivas and L. A. Mora-Tembre. 2022. UAV-Based characterization of tree-attributes and multispectral indices in an uneven-aged mixed conifer-broadleaf forest. Remote Sensing 14(12):2775. Doi: 10.3390/rs14122775. DOI: https://doi.org/10.3390/rs14122775
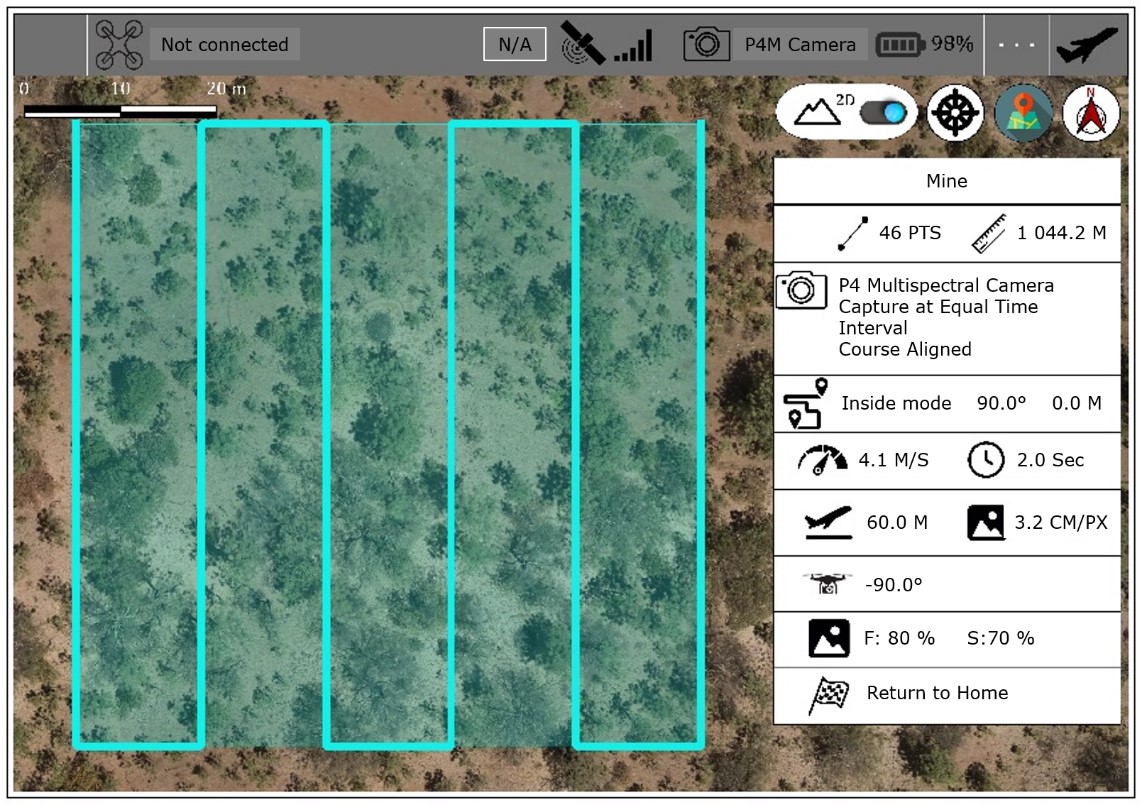
Descargas
Publicado
Cómo citar
Número
Sección
Licencia
Derechos de autor 2024 Revista Mexicana de Ciencias Forestales

Esta obra está bajo una licencia internacional Creative Commons Atribución-NoComercial 4.0.
Los autores que publiquen en la Revista Mexicana de Ciencias Forestales aceptan las siguientes condiciones:
De acuerdo con la legislación de derechos de autor, la Revista Mexicana de Ciencias Forestales reconoce y respeta el derecho moral de los autores, así como la titularidad del derecho patrimonial, el cual será cedido a la revista para su difusión en acceso abierto.
Todos los textos publicados por la Revista Mexicana de Ciencias Forestales –sin excepción– se distribuyen amparados bajo la licenciaCreative Commons 4.0 Atribución-No Comercial (CC BY-NC 4.0 Internacional), que permite a terceros utilizar lo publicado siempre que mencionen la autoría del trabajo y a la primera publicación en esta revista. (no permite el uso comercial)
Los autores pueden realizar otros acuerdos contractuales independientes y adicionales para la distribución no exclusiva de la versión del artículo publicado en la Revista Mexicana de Ciencias Forestales (por ejemplo, incluirlo en un repositorio institucional o darlo a conocer en otros medios en papel o electrónicos) siempre que indique clara y explícitamente que el trabajo se publicó por primera vez en la Revista Mexicana de Ciencias Forestales.
Para todo lo anterior, los autores deben remitir el formato de carta-cesión de la propiedad de los derechos de la primera publicación debidamente requisitado y firmado por los autores/as. Este formato debe ser remitido en archivo PDF al correo: editorial.forestal@inifap.gob.mx
Esta obra está bajo una licencia de Creative Commons Reconocimiento-No Comercial 4.0 Internacional.