Comparación de bases de datos climáticos en la modelación de distribución potencial de Pinus cembroides Zucc.
DOI:
https://doi.org/10.29298/rmcf.v14i79.1350Palabras clave:
distribucion de especies, datos climaticos, maxima entropia, modelos de distribucion, Pinus cembroides Zucc., variables bioclimaticasResumen
La distribución potencial de las poblaciones de Pinus cembroides depende de la variabilidad espacial y temporal de la temperatura y la precipitación. Dado el incremento en la disponibilidad de diferentes bases de datos climáticos en las últimas décadas, el objetivo del presente estudio fue evaluar el efecto de su variabilidad espacial y temporal en la modelación de la distribución potencial de P. cembroides. Se utilizó el algoritmo de Máxima Entropía (MaxEnt) para obtener la distribución potencial de P. cembroides a partir de los registros del Inventario Nacional Forestal y de Suelos y del Sistema Nacional de Información sobre Biodiversidad, con datos de cuatro fuentes de información climática. A pesar de las diferencias en la resolución espacial, se obtuvieron cuatro modelos confiables con valores de AUC cercanos a 0.8. La distribución de P. cembroides está limitada por la temperatura media de los trimestres más húmedo (Bio 8) y más seco (Bio 9). Los modelos de WorldClim v2.1 y SCM presentaron una mayor correlación entre la distribución de P. cembroides y las variables bioclimáticas seleccionadas. En los cuatro modelos, la especie registró una mayor probabilidad de ocurrencia (>70 %) en las sierras Madre Oriental y Occidental. Se concluye que son necesarias bases de datos con una resolución espacial de al menos 15 km2 para los estudios de distribución de P. cembroides. Este tipo de investigaciones deben considerarse un primer paso en la planeación y desarrollo de estrategias de manejo y conservación de la especie.
Descargas
Citas
Abdulwahab, U. A., E. Hammill and C. P. Hawkins. 2022. Choice of climate data affects the performance and interpretation of species distribution models. Ecological Modelling 471:110042. Doi: 10.1016/j.ecolmodel.2022.110042. DOI: https://doi.org/10.1016/j.ecolmodel.2022.110042
Aceves-Rangel, L. D., J. Méndez-Gonzáles, M. A. García-Aranda y J. A. Nájera-Luna. 2018. Distribución potencial de 20 especies de pinos en México. Agrociencia 52(7):1043-1057. https://www.scielo.org.mx/scielo.php?script=sci_arttext&pid=S1405-31952018000701043. (24 de febrero de 2023).
Aguirre G., J. and J. F. Duivenvoorden. 2010. Can we expect to protect threatened species in protected areas? A case study of the genus Pinus in Mexico. Revista Mexicana de Biodiversidad 81(3):875-882. https://www.scielo.org.mx/scielo.php?pid=S1870-34532010000300027&script=sci_abstract&tlng=en. (22 de febrero de 2023).
Antúnez, P., M. E. Suárez-Mota, C. Valenzuela-Encinas and F. Ruiz-Aquino. 2018. The potential distribution of tree species in three periods of time under a climate change scenario. Forests 9(10):628. Doi: 10.3390/f9100628. DOI: https://doi.org/10.3390/f9100628
Araújo, M. B., R. G. Pearson, W. Thuiller and M. Erhard. 2005. Validation of species-climate impact models under climate change. Global Change Biology 11(9):1504-1513. Doi: 10.1111/j.1365-2486.2005.01000.x. DOI: https://doi.org/10.1111/j.1365-2486.2005.01000.x
Austin, M. P. and K. P. Van Niel. 2011. Improving species distribution models for climate change studies: Variable selection and scale. Journal of Biogeography 38(1):1-8. Doi: 10.1111/j.1365-2699.2010.02416.x. DOI: https://doi.org/10.1111/j.1365-2699.2010.02416.x
Baker, D. J., A. J. Hartley, J. W. Pearce-Higgins, R. G. Jones and S. G. Willis. 2017. Neglected issues in using weather and climate information in ecology and biogeography. Diversity and Distributions 23(3):329-340. Doi: 10.1111/ddi.12527. DOI: https://doi.org/10.1111/ddi.12527
Belsley, D. A. 1991. Conditioning diagnostics: collinearity and weak data in regression. Wiley. Chichester, WS, United Kingdom. 396 p.
Bobrowski, M., J. Weidinger and U. Schickhoff. 2021. Is new always better? Frontiers in global climate datasets for modeling treeline species in the Himalayas. Atmosphere 12(5):543. Doi: 10.3390/atmos12050543. DOI: https://doi.org/10.3390/atmos12050543
Bucklin, D. N., M. Basille, A. M. Benscoter, L. A. Brandt, ... and J. I. Watling. 2015. Comparing species distribution models constructed with different subsets of environmental predictors. Diversity and Distributions 21(1):23-35. Doi: 10.1111/ddi.12247. DOI: https://doi.org/10.1111/ddi.12247
Carlón A., T., M. E. Mendoza, J. Villanueva D. and Y. Li. 2018. Climatic response of Pinus cembroides Zucc. radial growth in Sierra del Cubo, Guanajuato, Mexico. Trees 32(2):1387-1399. Doi: 10.1007/s00468-018-1720-1. DOI: https://doi.org/10.1007/s00468-018-1720-1
Cavazos, T., R. Luna-Niño, R. Cerezo-Mota, R. Fuentes-Franco, … and E. Valenzuela. 2020. Climatic trends and regional climate models intercomparison over the CORDEX-CAM (Central America, Caribbean and Mexico) domain. International Journal of Climatology 40(3):1396-1420. Doi: 10.1002/joc.6276. DOI: https://doi.org/10.1002/joc.6276
Choudhury, M. R., P. Deb, H. Singha, B. Chakdar and M. Medhi. 2016. Predicting the probable distribution and threat of invasive Mimosa diplotricha Suavalle and Mikania micrantha Kunth in a protected tropical grassland. Ecological Engineering 97:23-31. Doi: 10.1016/j.ecoleng.2016.07.018. DOI: https://doi.org/10.1016/j.ecoleng.2016.07.018
Comisión Nacional Forestal (Conafor). 2012. Inventario Nacional Forestal y de Suelos. Informe 2004-2009. Coordinación General de Planeación e Información, Gerencia de Inventario Forestal y Geomática y Conafor. Zapopan, Jal., México. 212 p. https://www.ccmss.org.mx/wp-content/uploads/2014/10/Inventario_nacional_forestal_y_de_suelos_informe_2004_-_2009_.pdf. (7 de julio de 2022).
Comisión Nacional para el Conocimiento y Uso de la Biodiversidad (Conabio). 2008. Catálogo de metadatos geográficos. http://geoportal.conabio.gob.mx/metadatos/doc/html/clima1mgw.html. (23 de octubre de 2023).
Comisión Nacional para el Conocimiento y Uso de la Biodiversidad (Conabio). 2021. Portal de Geoinformación, Sistema Nacional de Información sobre Biodiversidad (SNIB). Comisión Nacional para el Conocimiento y Uso de la Biodiversidad. http://www.conabio.gob.mx/informacion/gis/. (5 de agosto de 2022).
Connor, T., V. Hull, A. Viña, A. Shortridge, … and J. Liu. 2018. Effects of grain size and niche breadth on species distribution modeling. Ecography 41(8):1270-1282. Doi: 10.1111/ecog.03416. DOI: https://doi.org/10.1111/ecog.03416
Constante G., V., J. Villanueva D., J. Cerano P., E. H. Cornejo O. y S. Valencia M. 2009. Dendrocronología de Pinus cembroides Zucc. y reconstrucción de precipitación estacional para el sureste de Coahuila. Revista Ciencia Forestal en México 34(106):17-38. https://www.scielo.org.mx/scielo.php?script=sci_arttext&pid=S1405-35862009000200002. (22 de febrero de 2023).
Cuervo-Robayo, A. P., O. Téllez-Valdés, M. A. Gómez-Albores, C. S. Venegas-Barrera, J. Manjarrez and E. Martínez-Meyer. 2014. An update of high-resolution monthly climate surfaces for Mexico. International Journal of Climatology 34(7):2427-2437. Doi: 10.1002/joc.3848. DOI: https://doi.org/10.1002/joc.3848
Datta, A., O. Schweiger and I. Kühn. 2020. Origin of climatic data can determine the transferability of species distribution models. NeoBiota 59:61–76. Doi: 10.3897/neobiota.59.36299. DOI: https://doi.org/10.3897/neobiota.59.36299
Dormann, C. F., J. Elith, S. Bacher, C. Buchmann, … and S. Lautenbach. 2013. Collinearity: a review of methods to deal with it and a simulation study evaluating their performance. Ecography 36(1):27-46. Doi: 10.1111/j.1600-0587.2012.07348.x. DOI: https://doi.org/10.1111/j.1600-0587.2012.07348.x
Elith, J., S. J. Phillips, T. Hastie, M. Dudík, Y. E. Chee and C. J. Yates. 2011. A statistical explanation of MaxEnt for ecologists. Diversity and Distributions 17(1):43-57. Doi: 10.1111/j.1472-4642.2010.00725.x. DOI: https://doi.org/10.1111/j.1472-4642.2010.00725.x
Fick, S. E. and R. J. Hijmans. 2017. WorldClim 2: New 1-km spatial resolution climate surfaces for global land areas. International Journal of Climatology 37(12):4302-4315. Doi: 10.1002/joc.5086. DOI: https://doi.org/10.1002/joc.5086
García-Aranda, M. A., J. Méndez-González and J. Y. Hernández-Arizmendi. 2018. Potential distribution of Pinus cembroides, Pinus nelsonii and Pinus culminicola in northeastern Mexico. Ecosistemas y Recursos Agropecuarios 5(13):3-13. Doi: 10.19136/era.a5n13.1396. DOI: https://doi.org/10.19136/era.a5n13.1396
Guisan, A., A. Lehmann, S. Ferrier, M. Austin, … and T. Hastie. 2006. Making better biogeographical predictions of species’ distributions. Journal of Applied Ecology 43(3):386-392. Doi: 10.1111/j.1365-2664.2006.01164.x. DOI: https://doi.org/10.1111/j.1365-2664.2006.01164.x
Gutiérrez-García, J. V., D. A. Rodríguez-Trejo, A. Villanueva-Morales, S. García-Díaz y J. L. Romo-Lozano. 2015. Calidad del agua en la producción de Pinus cembroides Zucc. en vivero. Agrociencia 49(2):205-219. https://www.redalyc.org/articulo.oa?id=30236851008. (24 de febrero de 2023).
Harris, I., P. D. Jones, T. J. Osborn and D. H. Lister. 2014. Updated high-resolution grids of monthly climatic observations—the CRU TS3.10 Dataset. International Journal of Climatology 34(3):623-642. Doi: 10.1002/joc.3711. DOI: https://doi.org/10.1002/joc.3711
Herrera-Soto, G., M. González-Cásares, M. Pompa-García, J. J. Camarero and R. Solís-Moreno. 2018. Growth of Pinus cembroides Zucc. in response to hydroclimatic variability in four sites forming the species latitudinal and longitudinal distribution limits. Forests 7(7):440. Doi: https://doi.org/10.3390/f9070440. DOI: https://doi.org/10.3390/f9070440
Jiménez-Valverde, A., M. Rodríguez-Rey and P. Peña-Aguilera. 2021. Climate data source matters in species distribution modelling: the case of the Iberian Peninsula. Biodiversity and Conservation 30(1):67-84. Doi: 10.1007/s10531-020-02075-6. DOI: https://doi.org/10.1007/s10531-020-02075-6
Lembrechts, J. J., J. Lenoir, N. Roth, T. Hattab, A… and I. Nijs. 2019. Comparing temperature data sources for use in species distribution models: From in-situ logging to remote sensing. Global Ecology and Biogeography 28(11):1578-1596. Doi: 10.1111/geb.12974. DOI: https://doi.org/10.1111/geb.12974
Livneh, B., T. J. Bohn, D. W. Pierce, F. Munoz-Arriola, … and L. Brekke. 2015. A spatially comprehensive, hydrometeorological data set for Mexico, the U. S., and Southern Canada 1950-2013. Scientific Data 2:150042. Doi: 10.1038/sdata.2015.42. DOI: https://doi.org/10.1038/sdata.2015.42
Manzanilla-Quijada, G. E., E. J. Treviño-Garza, B. Vargas-Larreta, J. O. López-Martínez y J. M. Mata-Balderas. 2020. Áreas idóneas con potencial para la producción de semillas de Pinus chihuahuana Engelm. y Pinus leiophylla Schltdl. & Cham. en México. Botanical Sciences 98(2):305-316. Doi: 10.17129/botsci.2514. DOI: https://doi.org/10.17129/botsci.2514
Marino, J., M. Bennett, D. Cossios, A. Iriarte, ... and S. Walker. 2011. Bioclimatic constraints to Andean cat distribution: a modelling application for rare species. Diversity and Distributions 17(2):311-322. Doi: 10.1111/j.1472-4642.2011.00744.x. DOI: https://doi.org/10.1111/j.1472-4642.2011.00744.x
Nezer, O., S. Bar-David, T. Gueta and Y. Carmel. 2017. High-resolution species-distribution model based on systematic sampling and indirect observations. Biodiversity and Conservation 26(2):421-437. Doi: 10.1007/s10531-016-1251-2. DOI: https://doi.org/10.1007/s10531-016-1251-2
Pearson, R. G., C. J. Raxworthy, M. Nakamura and A. T. Peterson. 2007. Predicting species distributions from small numbers of occurrence records: a test case using cryptic geckos in Madagascar. Journal of Biogeography 34(1):102-117. Doi: 10.1111/j.1365-2699.2006.01594.x. DOI: https://doi.org/10.1111/j.1365-2699.2006.01594.x
Phillips, S. J. and M. Dudík. 2008. Modeling of species distributions with Maxent: new extensions and a comprehensive evaluation. Ecography 31(2):161-175. Doi: 10.1111/j.2007.0906-7590.05203.x. DOI: https://doi.org/10.1111/j.0906-7590.2008.5203.x
Phillips, S. J., R. P. Anderson and R. E. Schapire. 2006. Maximum entropy modeling of species geographic distributions. Ecological Modelling 190(3-4):231–259. Doi: 10.1016/j.ecolmodel.2005.03.026. DOI: https://doi.org/10.1016/j.ecolmodel.2005.03.026
Romero-Sánchez, M. E., A. González-Hernández, R. Pérez-Miranda, E. Velasco-Bautista y F. Moreno-Sánchez. 2017. Efecto del Cambio Climático a nivel local en la distribución potencial de cuatro especies forestales de la Cuenca Río-Bravo-San Juan, Coahuila, México. Agroproductividad 10(8):42-47. https://revista-agroproductividad.org/index.php/agroproductividad/article/view/1073. (22 de febrero de 2023).
Rzedowski, J. 1978. Vegetación de México. Limusa, S. A. México, D. F. México. 432 p.
Silva, L. D., E. B. de Azevedo, F. V. Reis, R. B. Elias and L. Silva. 2019. Limitations of species distribution models based on available climate change data: A case study in the Azorean forest. Forests 10(7):575. Doi: 10.3390/f10070575. DOI: https://doi.org/10.3390/f10070575
Stewart, S. B., M. Fedrigo, S. Kasel, S. H. Roxburgh, … and C. R. Nitschke. 2022. Predicting plant species distributions using climate-based model ensembles with corresponding measures of congruence and uncertainty. Diversity and Distributions 28(5):1105-1122. Doi: 10.1111/ddi.13515. DOI: https://doi.org/10.1111/ddi.13515
Téllez-Valdés, O., C. Miguel-Talonia, M. E. Suárez-Mota, R. X. Álvarez-Espino y M. M. Hernández-Moreno. 2019. Distribución potencial de las especies Pinaceae (Pinus) y Fagaceae (Quercus) de México. Informe final SNIB-CONABIO proyecto JM010. Facultad de Estudios Superiores Iztacala y Universidad Nacional Autónoma de México. Tlalnepantla de Reyes, Edo. Méx., México. 26 p. http://www.conabio.gob.mx/institucion/proyectos/resultados/InfJM010.pdf. (23 de octubre de 2022).
Vanuyytrecht, E., H. Wouters, J. Berckmans and K. De Ridde. 2021. Global bioclimatic indicators from 1979 to 2018 derived from reanalysis. Product User Guide. Copernicus Climate Change Service. Reading, WBK, United Kingdom. 40 p. https://datastore.copernicus-climate.eu/documents/sis-biodiversity/C3S_D427.3.1.1_Product_user_guide-Bioclimatic_indicators_ERA5_v2.3.pdf. (23 de octubre de 2023).
Watling, J. I., R. J. Fletcher, C. Speroterra, D. N. Bucklin, … and F. J. Mazzotti. 2014. Assessing effects of variation in global climate data sets on spatial predictions from climate envelope models. Journal of Fish and Wildlife Management 5(1):14-25. Doi: 10.3996/072012-JFWM-056. DOI: https://doi.org/10.3996/072012-JFWM-056
Zhang, Y., J. Tang, G. Ren, K. Zhao and X. Wang. 2021. Global potential distribution prediction of Xanthium italicum based on Maxent model. Scientific Reports 11(1):16545. Doi: 10.1038/s41598-021-96041-z. DOI: https://doi.org/10.1038/s41598-021-96041-z
Zhu, C. and D. P. Lettenmaier. 2007. Long-term climate and derived surface hydrology and energy flux data for Mexico: 1925-2004. Journal of Climate 20(9):1936-1946. Doi: 10.1175/JCLI4086.1. DOI: https://doi.org/10.1175/JCLI4086.1
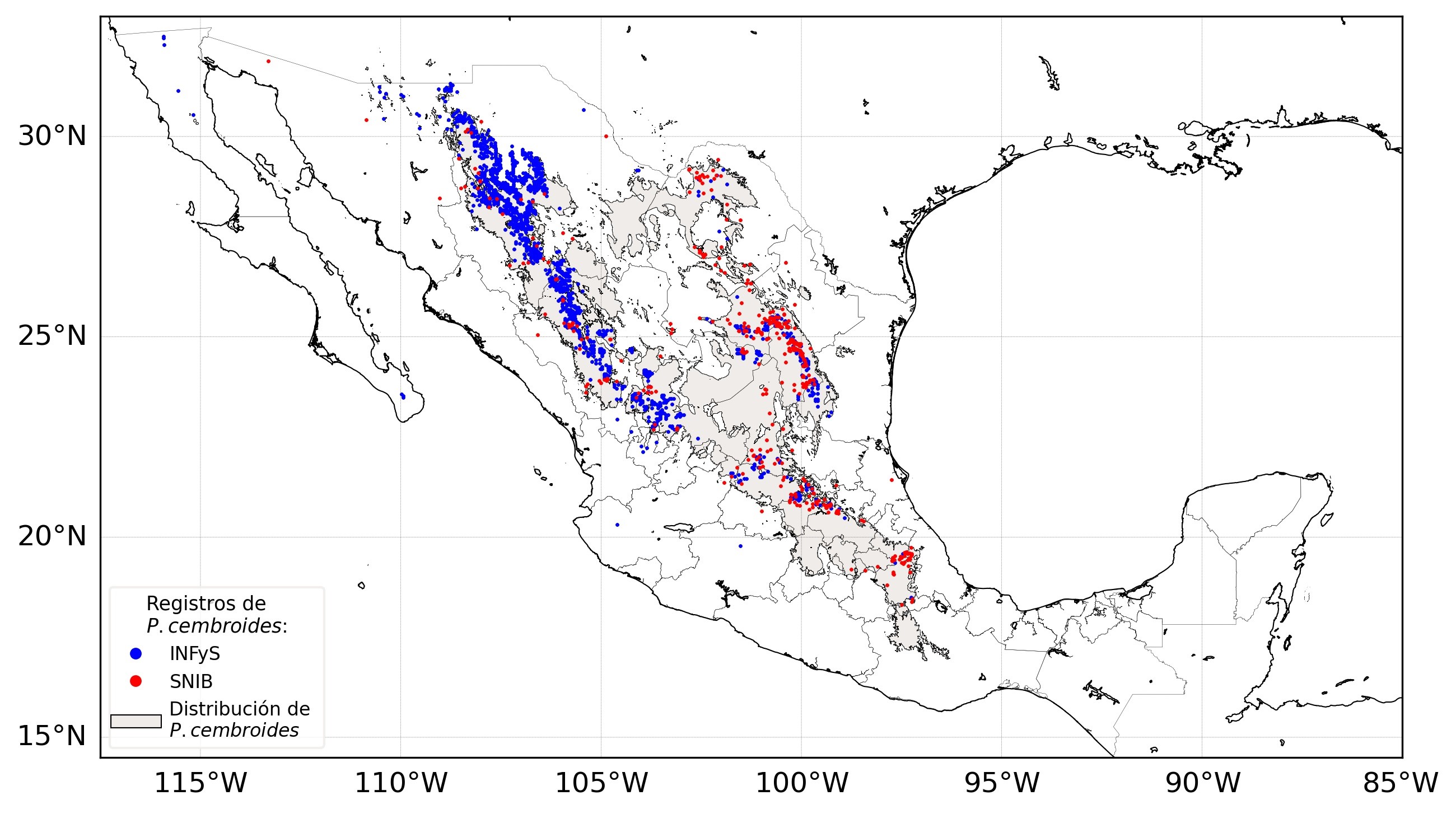
Descargas
Publicado
Cómo citar
Número
Sección
Licencia
Derechos de autor 2023 Revista Mexicana de Ciencias Forestales

Esta obra está bajo una licencia internacional Creative Commons Atribución-NoComercial 4.0.
Los autores que publiquen en la Revista Mexicana de Ciencias Forestales aceptan las siguientes condiciones:
De acuerdo con la legislación de derechos de autor, la Revista Mexicana de Ciencias Forestales reconoce y respeta el derecho moral de los autores, así como la titularidad del derecho patrimonial, el cual será cedido a la revista para su difusión en acceso abierto.
Todos los textos publicados por la Revista Mexicana de Ciencias Forestales –sin excepción– se distribuyen amparados bajo la licenciaCreative Commons 4.0 Atribución-No Comercial (CC BY-NC 4.0 Internacional), que permite a terceros utilizar lo publicado siempre que mencionen la autoría del trabajo y a la primera publicación en esta revista. (no permite el uso comercial)
Los autores pueden realizar otros acuerdos contractuales independientes y adicionales para la distribución no exclusiva de la versión del artículo publicado en la Revista Mexicana de Ciencias Forestales (por ejemplo, incluirlo en un repositorio institucional o darlo a conocer en otros medios en papel o electrónicos) siempre que indique clara y explícitamente que el trabajo se publicó por primera vez en la Revista Mexicana de Ciencias Forestales.
Para todo lo anterior, los autores deben remitir el formato de carta-cesión de la propiedad de los derechos de la primera publicación debidamente requisitado y firmado por los autores/as. Este formato debe ser remitido en archivo PDF al correo: editorial.forestal@inifap.gob.mx
Esta obra está bajo una licencia de Creative Commons Reconocimiento-No Comercial 4.0 Internacional.