Clasificación de uso del suelo y vegetación con redes neuronales convolucionales
DOI:
https://doi.org/10.29298/rmcf.v13i74.1269Palabras clave:
aprendizaje de máquina, clasificación automática, imágenes Sentinel-2, cuenca Atoyac-Salado, inteligencia artificial, sensores remotosResumen
La clasificación de uso del suelo y vegetación es un ejercicio complejo y difícil de realizar con métodos tradicionales, por lo que los modelos de aprendizaje profundo son una alternativa para su aplicación debido a que son altamente capaces de aprender esta semántica compleja, lo que hace plausible su aplicación en la identificación automática de usos del suelo y vegetación, a partir de patrones espacio-temporales extraídos de su apariencia. El objetivo del presente estudio fue proponer y evaluar un modelo de red neuronal convolucional de aprendizaje profundo para la clasificación de 22 clases distintas de cobertura y uso del suelo ubicadas en la cuenca río Atoyac-Salado. El modelo propuesto se entrenó utilizando datos digitales capturados en 2021 por el satélite Sentinel 2; se aplicó una combinación diferente de hiperparámetros, en la cual la precisión del modelo depende del optimizador, la función de activación, el tamaño del filtro, la tasa de aprendizaje y el tamaño del lote. Los resultados proporcionaron una precisión de 84.57 % para el conjunto de datos. Para reducir el sobreajuste se empleó el método de regularización denominado dropout, que resultó ser muy eficaz. Se comprobó con suficiente precisión que el aprendizaje profundo con redes neuronales convolucionales identifica patrones en los datos de la reflectancia captada por las imágenes del satélite Sentinel 2 para la clasificación el uso de suelo y vegetación en áreas con una dificultad intrínseca en la cuenca del río Atoyac-Salado
Descargas
Citas
Bhosle, K. and Musande, V. 2019. Evaluation of Deep Learning CNN Model for LULC Classification and Crop Identification Using Hyperspectral Remote Sensing Images. India. Journal of the Indian Society of Remote Sensing, 47(11):1949–1958. https://doi.org/10.1007/s12524-019-01041-2 DOI: https://doi.org/10.1007/s12524-019-01041-2
Bocco, M.; Ovando, G.; Sayago, S. and Willington, E. 2007. Neural Network Model for Land Cover Classification from Satellite Images. Arg. Agricultura Técnica, 67(4): 414–421. https://doi.org/10.4067/S0365-28072007000400009 DOI: https://doi.org/10.4067/S0365-28072007000400009
Borràs, J.; Delegido, J.; Pezzola, A.; Pereira, M.; Morassi, G. and Camps-Valls, G. 2017. Clasificación de usos del suelo a partir de imágenes Sentinel-2. Revista de Teledetección, 2017(48):55. https://doi.org/10.4995/raet.2017.7133 DOI: https://doi.org/10.4995/raet.2017.7133
Chen, Y.; Jiang, H.; Li, C.; Jia, X. and Ghamisi, P. 2016. Deep Feature Extraction and Classification of Hyperspectral Images Based on Convolutional Neural Networks. China. IEEE Transactions on Geoscience and Remote Sensing, 54(10):6232–6251. https://doi.org/10.1109/TGRS.2016.2584107 DOI: https://doi.org/10.1109/TGRS.2016.2584107
Congalton, R. G. and Green, K. 2009. Sample Design Considerations. United States of America in C. Press (Ed.), Assessing the Accuracy of Remotely Sensed Data: Principles and Practices, Second Edition. (63–83). https://doi.org/10.1201/9781420055139.ch5 DOI: https://doi.org/10.1201/9781420055139.ch5
DOF. 2017. Acuerdo por el que se dan a conocer los resultados del estudio técnico de las aguas nacionales superficiales en las cuencas hidrológicas Río Papagayo 1, Río Petaquillas, Río Omitlán, Río Papagayo 2, Río Papagayo 3, Río Papagayo 4, Río Nexpa 1 y 2. México. Diario Oficial de La Federación. https://dof.gob.mx/nota_detalle.php?codigo=5496053yfecha=04/09/2017
Drusch, M.; Del Bello, U.; Carlier, S.; Colin, O.; Fernandez, V.; Gascon, F.; Hoersch, B.; Isola, C.; Laberinti, P.; Martimort, P.; Meygret, A.; Spoto, F.; Sy, O.; Marchese, F. and Bargellini, P. 2012. Sentinel-2: ESA’s Optical High-Resolution Mission for GMES Operational Services. Netherlands. Remote Sensing of Environment, 120:25–36. https://doi.org/10.1016/j.rse.2011.11.026 DOI: https://doi.org/10.1016/j.rse.2011.11.026
Gascon, F.; Bouzinac, C.; Thépaut, O.; Jung, M.; Francesconi, B.; Louis, J.; Lonjou, V.; Lafrance, B.; Massera, S.; Gaudel-Vacaresse, A.; Languille, F.; Alhammoud, B.; Viallefont, F.; Pflug, B.; Bieniarz, J.; Clerc, S.; Pessiot, L.; Trémas, T.; Cadau, E. and Fernandez, V. Copernicus Sentinel-2A Calibration and Products Validation Status. France. Remote Sensing, 9(6):584. https://doi.org/10.3390/rs9060584 DOI: https://doi.org/10.3390/rs9060584
Gnip, P.; Vokorokos, L. and Drotár, P. 2021. Selective oversampling approach for strongly imbalanced data. Slovak Republic. PeerJ Computer Science, 7, e604. https://doi.org/10.7717/peerj-cs.604 DOI: https://doi.org/10.7717/peerj-cs.604
Hermosilla, T.; Wulder, M. A.; White, J. C. and Coops, N. C. 2022. Land cover classification in an era of big and open data: Optimizing localized implementation and training data selection to improve mapping outcomes. Remote Sensing of Environment, 268. https://doi.org/10.1016/j.rse.2021.112780 DOI: https://doi.org/10.1016/j.rse.2021.112780
Hu, F.; Xia, G. S.; Hu, J. and Zhang, L. 2018. A deep convolution neural network method for land cover mapping: A case study of Qinhuangdao, China. China. Remote Sensing, 10(12):1–17. https://doi.org/10.3390/rs10122053 DOI: https://doi.org/10.3390/rs10122053
INEGI. 2017. Guía para la interpretación de cartografía: uso del suelo y vegetación. México. Instituto Nacional de Estadística y Geografía, 204
Kingma, D. P. and Ba, J. 2014. Adam: A Method for Stochastic Optimization. 3rd International Conference on Learning Representations, ICLR, 1–15. http://arxiv.org/abs/1412.6980
Krizhevsky, A.; Sutskever, I. and Hinton, G. E. 2012. ImageNet Classification with Deep Convolutional Neural Networks. Canada. Advances in Neural Information Processing Systems, 25. http://www.cs.toronto.edu/~fritz/absps/imagenet.pdf
Lawley, V.; Lewis, M.; Clarke, K. and Ostendorf, B. 2016. Site-based and remote sensing methods for monitoring indicators of vegetation condition: An Australian review. Ecological Indicators, 60:1273–1283. https://doi.org/10.1016/j.ecolind.2015.03.021 DOI: https://doi.org/10.1016/j.ecolind.2015.03.021
Liu, R.; Yang, X.; Xu, C.; Wei, L. and Zeng, X. 2022. Comparative Study of Convolutional Neural Network and Conventional Machine Learning Methods for Landslide Susceptibility Mapping. China. Remote Sensing, 14(2):321. https://doi.org/10.3390/rs14020321 DOI: https://doi.org/10.3390/rs14020321
Macedo, A.; Pajares, G. y Santos, M. 2010. Clasificación no supervisada con imágenes a color de cobertura terrestre. México. Agrociencia, 44(6):711–722. https://www.redalyc.org/articulo.oa?id=30215554009
Mas, J. F.; Velázquez, A. y Couturier, S. 2009. La evaluación de los cambios de cobertura / uso del suelo en la República Mexicana. México. Investigación Ambiental, 1(1):23–39
Meyer, W. and Turner II, B. 1992. Human Population Growth and Global Land-Use/Cover Change. United States of America. Annual Review of Ecology and Systematics, 23:39–61 DOI: https://doi.org/10.1146/annurev.es.23.110192.000351
Najafabadi, M. M.; Villanustre, F.; Khoshgoftaar, T. M.; Seliya, N.; Wald, R. and Muharemagic, E. 2015. Deep learning applications and challenges in big data analytics. United States of America. Journal of Big Data, 2(1):1–21. https://doi.org/10.1186/s40537-014-0007-7 DOI: https://doi.org/10.1186/s40537-014-0007-7
Olofsson, P.; Foody, G. M.; Herold, M.; Stehman, S. V.; Woodcock, C. E. and Wulder, M. A. 2014. Good practices for estimating area and assessing accuracy of land change. Remote Sensing of Environment. Elsevier Inc. 148:42-57 https://doi.org/10.1016/j.rse.2014.02.015 DOI: https://doi.org/10.1016/j.rse.2014.02.015
Patel, S. K.; Verma, P. and Singh, G. S. 2019. Agricultural growth and land use land cover change in peri-urban India. Environmental Monitoring and Assessment 191(9):600. https://doi.org/10.1007/s10661-019-7736-1 DOI: https://doi.org/10.1007/s10661-019-7736-1
Paz, F.; Romero, V. M.; Argumedo-Espinoza, J. A.; Bolaños, M.; de Jong, B.; de la Cruz, J. C. y Velázquez, A. 2019. Dinámica Del Uso Del Suelo Y Vegetación. México. In Estado del Ciclo del Carbono en México, Agenda Azul y Verde (Vol. 23, Issue June. 529–572)
Pellikka, P. K. E.; Clark, B. J. F.; Gosa, A. G.; Himberg, N.; Hurskainen, P.; Maeda, E.; Mwang’ombe, J.; Omoro, L. M. A. and Siljander, M. 2013. Agricultural Expansion and Its Consequences in the Taita Hills, Kenya. In Developments in Earth Surface Processes. Elsevier. 16:65–179. https://doi.org/10.1016/B978-0-444-59559-1.00013-X DOI: https://doi.org/10.1016/B978-0-444-59559-1.00013-X
Ponce, J. C.; Torres, A.; Quezada, F. S.; Silva, A.; Martínez, E. U.; Casali, F. A.; Scheihing, E.; Túpac, Y. J.; Torres, M. D.; Ornelas, F. J.; Hernández, J. A.; Zavala, C.; Vakhni, N. y Pedreño, O. 2014. Inteligencia Artificial. In Iniciativa Latinoamericana de Libros de Texto Abiertos (Vol. 1)
Rousset, G.; Despinoy, M.; Schindler, K. and Mangeas, M. 2021. Assessment of deep learning techniques for land use land cover classification in southern new caledonia. France. Remote Sensing, 13(12):1–22. https://doi.org/10.3390/rs13122257 DOI: https://doi.org/10.3390/rs13122257
Sarker, I. H. 2021. Machine Learning: Algorithms, Real-World Applications and Research Directions. Australia. SN Computer Science, 2(3)160. https://doi.org/10.1007/s42979-021-00592-x DOI: https://doi.org/10.1007/s42979-021-00592-x
Shalev, S. and Ben, S. 2014. Understanding machine learning: From theory to algorithms. United States of America. In Understanding Machine Learning: From Theory to Algorithms. https://doi.org/10.1017/CBO9781107298019 DOI: https://doi.org/10.1017/CBO9781107298019
Srivastava, N.; Hinton, G.; Krizhevsky, A.; Sutskever, I. and Salakhutdinov, R. 2014. Dropout: A Simple Way to Prevent Neural Networks from Overfitting. Canada, Journal of Machine Learning Research, 15:1929–1958
Suárez, A. S.; Jiménez, A. F.; Castro, M. y Cruz, A. A. 2017. Clasificación y mapeo automático de coberturas del suelo en imágenes satelitales utilizando Redes Neuronales Convolucionales. Colombia. Orinoquia, 21(1 Sup):64–75. https://doi.org/10.22579/20112629.432 DOI: https://doi.org/10.22579/20112629.432
Theodoridis, S. 2015. Introduction. Netherlands. In Machine Learning (1–8). Elsevier. https://doi.org/10.1016/B978-0-12-801522-3.00001-X DOI: https://doi.org/10.1016/B978-0-12-801522-3.00001-X
Verona, P.; Cristina, I. y García, A. 2016. Una revisión sobre aprendizaje no supervisado de métricas de distancia. Cuba. Revista Cubana de Ciencias Informáticas, 10(4):43–67
Vinet, L. and Zhedanov, A. 2011. A ‘missing’ family of classical orthogonal polynomials. Canada. Journal of Physics A: Mathematical and Theoretical, 44(8). https://doi.org/10.1088/1751-8113/44/8/085201 DOI: https://doi.org/10.1088/1751-8113/44/8/085201
Xie, Z.; Chen, Y.; Lu, D.; Li, G. and Chen, E. 2019. Classification of Land Cover, Forest, and Tree Species Classes with ZiYuan-3 Multispectral and Stereo Data. Remote Sensing, 11(2):164. http://dx.doi.org/10.3390/rs11020164 DOI: https://doi.org/10.3390/rs11020164
Xie, G.; Shangguan, A.; Fei, R.; Ji, W.; Ma, W. and Hei, X. 2020. Motion trajectory prediction based on a CNN-LSTM sequential model. Science China Information Sciences, 63(11):1-21. https://doi.org/10.1007/s11432-019-2761-y DOI: https://doi.org/10.1007/s11432-019-2761-y
Yeturu, K. 2020. Machine learning algorithms, applications, and practices in data science. India. In Handbook of Statistics (1st ed., Vol. 43, 81–206). Elsevier B.V. https://doi.org/10.1016/bs.host.2020.01.002 DOI: https://doi.org/10.1016/bs.host.2020.01.002
Zhang, C.; Sargent, I.; Pan, X.; Li, H.; Gardiner, A.; Hare, J. and Atkinson, P. M. 2019. Joint Deep Learning for land cover and land use classification. United Kindom. Remote Sensing of Environment, 221:173–187. https://doi.org/10.1016/j.rse.2018.11.014 DOI: https://doi.org/10.1016/j.rse.2018.11.014
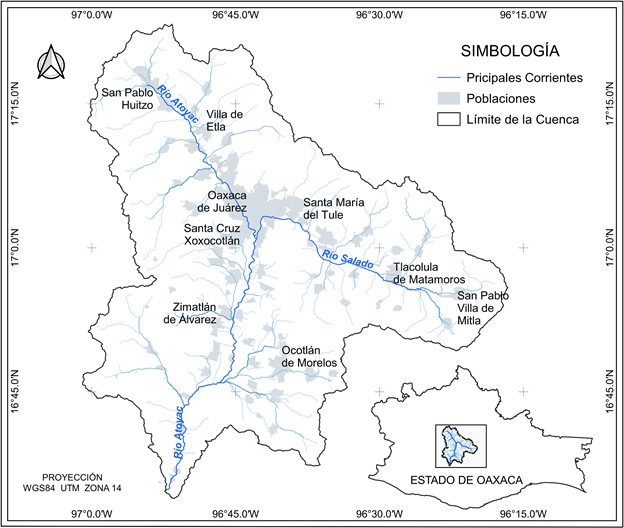
Descargas
Publicado
Cómo citar
Número
Sección
Licencia
Derechos de autor 2022 Revista Mexicana de Ciencias Forestales

Esta obra está bajo una licencia internacional Creative Commons Atribución-NoComercial 4.0.
Los autores que publiquen en la Revista Mexicana de Ciencias Forestales aceptan las siguientes condiciones:
De acuerdo con la legislación de derechos de autor, la Revista Mexicana de Ciencias Forestales reconoce y respeta el derecho moral de los autores, así como la titularidad del derecho patrimonial, el cual será cedido a la revista para su difusión en acceso abierto.
Todos los textos publicados por la Revista Mexicana de Ciencias Forestales –sin excepción– se distribuyen amparados bajo la licenciaCreative Commons 4.0 Atribución-No Comercial (CC BY-NC 4.0 Internacional), que permite a terceros utilizar lo publicado siempre que mencionen la autoría del trabajo y a la primera publicación en esta revista. (no permite el uso comercial)
Los autores pueden realizar otros acuerdos contractuales independientes y adicionales para la distribución no exclusiva de la versión del artículo publicado en la Revista Mexicana de Ciencias Forestales (por ejemplo, incluirlo en un repositorio institucional o darlo a conocer en otros medios en papel o electrónicos) siempre que indique clara y explícitamente que el trabajo se publicó por primera vez en la Revista Mexicana de Ciencias Forestales.
Para todo lo anterior, los autores deben remitir el formato de carta-cesión de la propiedad de los derechos de la primera publicación debidamente requisitado y firmado por los autores/as. Este formato debe ser remitido en archivo PDF al correo: editorial.forestal@inifap.gob.mx
Esta obra está bajo una licencia de Creative Commons Reconocimiento-No Comercial 4.0 Internacional.